top of page
Research Projects
3D Multi-Object Tracking
3D multi-object tracking is a key problem for autonomous vehicles, required to perform well-informed motion planning in dynamic environments. Particularly for densely occupied scenes, associating existing tracks to new detections remains challenging as existing systems tend to omit critical contextual information
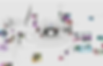
Drone Landing
UAV/UGV teams show strong promise for a range of applications, thanks to their complementary capabilities of maneuverability and endurance. In this project, we demonstrate some of the first automated docking of drones on moving ground vehicles, and push the state of the art in quadrotor aerodynamic modeling, pose estimation, and precision control.
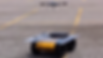
bottom of page